Joseph Salmon
Machine learning & data science
I am a statistician and an applied mathematician, with a strong interest in machine learning, optimization and data science.
Since Oct. 2024, I have been a senior researcher at Inria (team Iroko).
In terms of applications, I focus on citizen science, crowdsourcing and high dimensional statistics. I am also in charge of the doctoral program "Statistics and Data Science" (EDI2S at Université de Montpellier).
Short bio
From 2018 to 2024, I was a full professor at Université de Montpellier and a Junior member of the Institut Universitaire de France (IUF), from 2021 to 2024. For the spring and summer quarters 2018, I was a visiting assistant professor at UW, Statistics department. From 2012 to 2018 I was an assistant professor at Telecom Paris and an associate member at INRIA Parietal Team (now Mind Team). Back in 2011-2012, I was a post-doctoral Associate at Duke university working with Rebecca Willett. In 2010, I finished my Ph.D. in statistics and image processing under the supervision of Dominique Picard and Erwan Le Pennec at the Laboratoire de Probabilités et de Modélisation Aléatoire, now LPSM, in Université Paris Diderot.
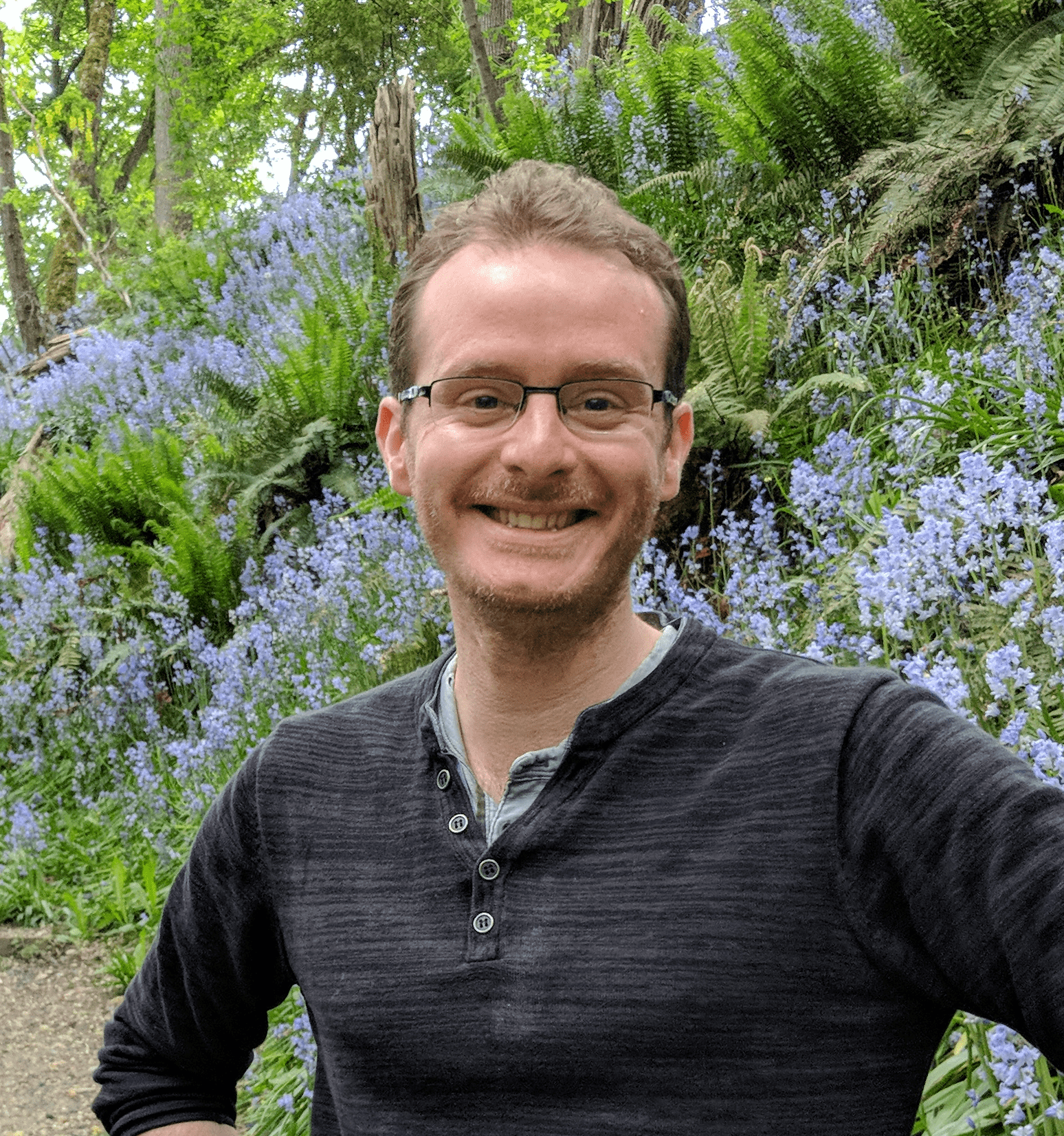
Recent Publications
-
peerannot: A framework for label aggregation in crowdsourced datasets
JDS 2024 - 55es Journées de Statistique, 2024
PDF Code -
Local linear convergence of proximal coordinate descent
algorithm
Optimization Letter, 2024
PDF -
Identify ambiguous tasks combining crowdsourced labels by weighting Areas Under the Margin
TMLR, 2024
PDF Code Dataset -
Peerannot: classification for crowd-sourced image datasets with Python
Computo, 2024
PDF Code -
Cooperative learning of Pl@ntNet's Artificial Intelligence algorithm: how does it work and how can we improve it?
, 2024
PDF Code Dataset -
Weighted majority vote using Shapley values in crowdsourcing
CAp 2024 - Conférence sur l'Apprentissage Automatique, 2024
PDF Code
A full list of publications can be found here.
News
- Sept. 2024: I’ve joined Inria as a senior researcher (Team: Iroko, Montpellier)
- Sept. 2023: ANR VITE (PI: B. Thirion, theme: variable importance / explainability) accepted.
- April 2023: STATLEARN 2023 in Montpellier
- Nov. 2022: https://ml4lifesciences.sciencesconf.org/
- March 2022: visitor at the Simons Institute for the Theory of Computing
- July 2021: IUF Nomination (junior member): https://www.iufrance.fr/detail-de-lactualite/247.html
- Nov. 2020: Launching ML-MTP Machine Learning in Montpellier, Theory & Practice.
- Dec. 2019: The ANR AI chair proposal CaMeLOt (CooperAtive MachinE Learning and OpTimization) has been selected.
- May 2019 : Workshop in Montpellier Graph signals : learning and optimization perspectives
Contact
If you want to connect please reach out via email joseph.salmon@inria.fr or Mastodon.
You can also write or visit me:
IMAG, c.c. 051
Université de Montpellier
Place Eugène Bataillon
34095 Montpellier Cedex 5
(office 415, building 9)